AI Ethics has a clickbait problem [Thoughts]
How a crucial aspect of AI has become a hotbed for bad faith discussions and misinformation
Hey, it’s Devansh 👋👋
Thoughts is a series on AI Made Simple. In issues of Thoughts, I will share interesting developments/debates in AI, go over my first impressions, and their implications. These will not be as technically deep as my usual ML research/concept breakdowns. Ideas that stand out a lot will then be expanded into further breakdowns. These posts are meant to invite discussions and ideas, so don’t be shy about sharing what you think.
If you’d like to support my writing, consider becoming a premium subscriber to my sister publication Tech Made Simple to support my crippling chocolate milk addiction. Use the button below for a discount.
p.s. you can learn more about the paid plan here. If your company is looking looking for software/tech consulting- my company is open to helping more clients. We help with everything- from staffing to consulting, all the way to end to website/application development. Message me using LinkedIn, by replying to this email, or on the social media links at the end of the article to discuss your needs and see if you’d be a good match.
AI Ethics is one of the most important crucial elements of AI. Most of my content focuses on how to build high-performing AI products, teams, and systems. However, when all is said and done, foreseeing and evaluating the downstream effects of the aforementioned products is as important as the engineering. Evaluating the products from an ethics perspective is critical in uncovering the underlying inductive biases present in our systems.
Unfortunately, much of the AI Ethics community has recently adopted the main-stream media playbook towards discussing AI Safety and Ethics- clickbait titles, bad-faith arguments, and ignoring the real issues in favor of sensationalist narratives. In this article, we will be going over some notable examples, why they are off, and why this ultimately harms the field of AI Safety.
Using a recipe bot to wage biological warfare
This was the incident that inspired this article. To those of you that aren’t familiar, a recipe bot in New Zealand gained a lot of attention when it recommended a special recipe for refreshing drink. The only problem, following the recipe would create chlorine gas, a very poisnous substance (it was used in WW1 to kill lots of soldiers). Naturally, this had mainstream media and a lot of the AI Safety folk frothing. Here was a clear case of AI harm, proof that all the societal level risks that the cult of AI Doomerism had been warning us about was true all along.
A look at reality paints a very different picture. While it is true that the reciple bot did recommend some very unhealthy recipes, this only happened when it was prompted to do so. The bot simply created recipes based on the recipes that users input into it. Give it recipes like cyanide and bleach, and the output will poison you. As with the other cases of AI harm discussed on this newsletter, the problem is not with the AI tool, but it’s misuse. Ofcourse, since many of the outraged folk only bothered skimming the headlines, they missed this crucial lil detail and continued to rage against the machine.
The recipe bot does have some interesting lessons, even from an AI Safety perspective. Most notably, it should be clear that LLMs lack discernment and judgement, which limits their ‘intelligence’. They have a map of the world that is fundamentally different to us, and thus should be evaluated and used differently. Human-like intelligence is a fun little sci-fi trope, but should be left there. Just because modern AI tools seem like they can do something, doesn’t mean that they can actually do these tasks well. Before trying to axe people and replace them with AI, it is prudent to actually evaluate what these models do well and what they struggle with. When it comes to fuzzy tasks like employee evaluation and customer service, AI may not be your best bet, no matter how competent these chatbots seem.
Instead of focusing on these learnings, our posse of internet intellectuals chose to resume their song and dance of how your grandma is a few misguided prompts away from becoming the next Orochimaru. Go figure.
However, common discourse is far from the only place where we see these bad-faith discussions occur. Let’s now look at a very high-profile academic publication that relied on bad-faith framing to generate more clicks.
Twitter and Male Gaze
If you have used Twitter or other social media, you will be familiar with image previews. When images are too big to be loaded, social media platforms will only display a small portion of them. Interested users can choose to click on the image preview to view the whole thing. AI is used to select which part of the image is best as a preview (for max engagement).
Twitter users noticed a very interesting phenomenon- for pictures of women, the preview would often cut out their faces out, and zoom in on their bodies. Given the historically high sexualization of women in media and tech being largely male dominated, it was not an unreasonable conclusion that AI had developed a male-gaze of it’s own. This lead to a lot of investigation into the topic. Enter the paper- Auditing saliency cropping algorithms.
The authors of this paper of this paper looked into whether AI was sexualizing women. Their abstract had this to say- In doing so, we present the first formal empirical study which suggests that the worry of a male-gazelike image cropping phenomenon on Twitter is not at all far-fetched and it does occur with worryingly high prevalence rates in real-world full-body single-female-subject images shot with logo-littered backdrops. They even go onto refer to this as MGL (male gaze like). However, a look into their own paper reveals a very different conclusion. Take a look at their investigation into the areas that the AI assigns the most importance to-
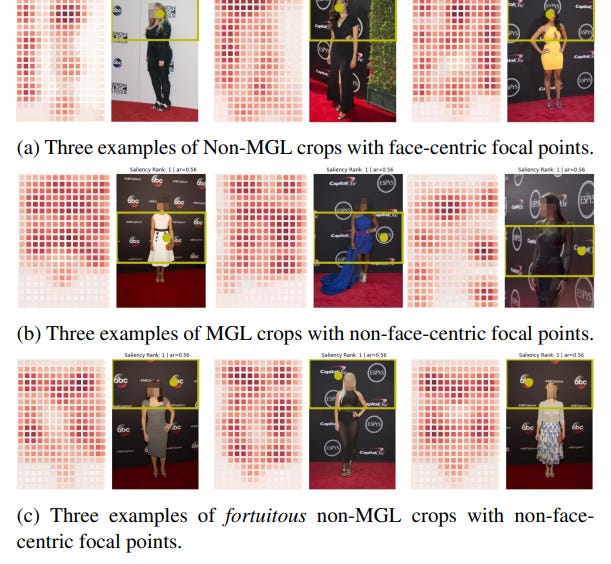
Notice any patterns? If we look at what is most consistent across images, one pattern pops up- the AI consistently ranks the corporate logos very highly. This makes sense, since Twitter as a platform is catered towards advertisers. Rather than a male gaze, the AI seems to be exhibit a consumerist gaze. Here is what the authors had to say-
In Figure 3b, we see how the focal point mapped to either the fashion accessory worn by the celebrity (left-most image) or the event logo (the ESPYs logo in the middle image) or the corporate logos (the Capital One logo in the right-most image) in the background which resulted in MGL artifacts in the final cropped image. In Figure 3c, we present examples of cases where a benign crop (free of MGL artifacts) emerged out of lucky serendipity where the focal point was not face-centric but was actually located on a background event or corporate logo(s), but the logo coincidentally happened to be located near the face or the top-half of the image thereby resulting in a final crop that gives the appearance of a face-centric crop.
However, the authors failed to mention this in the abstract and continued to use the term MGL. I don’t think I need to spend pages convincing you that this deliberate misdirection is extremely bad-faith and a clear indication that the authors were trying to bank on outrage to draw get some clicks. I know off many people who simply read the abstract and based their conclusions from that, instead of looking through the research. In this case, that would completely change the outcomes and learnings from the publications.
Once again, such click-bait harms much more than it helps. While it may get some views, people are more likely to dismiss the work once they realize the sleight of hand occuring here. And bad-actors might even try to use such studies to dismiss further investigation into sexual and racial biases present in systems (which will worsen these inequalities and cause serious damage to certain groups).
Police departments use predictive algorithms to strategize about where to send their ranks. Law enforcement agencies use face recognition systems to help identify suspects. These practices have garnered well-deserved scrutiny for whether they in fact improve safety or simply perpetuate existing inequities. Researchers and civil rights advocates, for example, have repeatedly demonstrated that face recognition systems can fail spectacularly, particularly for dark-skinned individuals—even mistaking members of Congress for convicted criminals.
Actions have consequences. These people drumming up all this outrage are diverting from very real issues that need our attention. The people engaged in this clickbair are crying wolf. Hate to break it to y’all, but the wolves are already here and they are eating the cripples. We just don’t hear the screams because we’re too busy listening to this outrage machine. The sad part is that when the harm takes place, these outrage merchants will turn right around wave around an “I told you so”, even though it was their constant exagerrations that distracted from the issues to begin with.
AI Doomers and AI Ethics Clickbait
To a degree, I can empathise with the origins with the AI Ethics Clickbait. It is an over correction because Silicon Valley VCs are doing really dumb stuff. If the dominant narrative in the media is that AI is God’s Gift to Humanity, then sometimes screaming in the opposite direction might seem like the only way to get the conversation about important topics going.
Bt ultimately, both positive hype and doomerism are equally harmful. Both are noise that lead to reckless adoption, focusing on the wrong issues, and ultimately worsen lives for most people (while profiting a select few). A while back, I covered the lucrative business of AI Hype, where I covered how both positive and negative hype ultimately operated on the same business model- generate anxiety about AI, sell you the solution. This approach relies on spreding misinformation and getting people caught up in the hype cycle. Below is a message I received after that piece. While the doomers might claim that they want to stop harm from AI, you will clearly see how their sensationalism causes real harm to the mental health of people. All for their popularity/gain.
I’ll sign off here, because there’s nothing I can say that the message doesn’t already say.
If any of you would like to work on this topic, feel free to reach out to me. If you’re looking for AI Consultancy, Software Engineering implementation, or more- my company, SVAM, helps clients in many ways: app development, strategy consulting, and staffing. Feel free to reach out and share your needs, and we can work something out.
That is it for this piece. I appreciate your time. As always, if you’re interested in working with me or checking out my other work, my links will be at the end of this email/post. If you like my writing, I would really appreciate an anonymous testimonial. You can drop it here. And if you found value in this write-up, I would appreciate you sharing it with more people. It is word-of-mouth referrals like yours that help me grow.
Reach out to me
Use the links below to check out my other content, learn more about tutoring, reach out to me about projects, or just to say hi.
Small Snippets about Tech, AI and Machine Learning over here
AI Newsletter- https://artificialintelligencemadesimple.substack.com/
My grandma’s favorite Tech Newsletter- https://codinginterviewsmadesimple.substack.com/
Check out my other articles on Medium. : https://rb.gy/zn1aiu
My YouTube: https://rb.gy/88iwdd
Reach out to me on LinkedIn. Let’s connect: https://rb.gy/m5ok2y
My Instagram: https://rb.gy/gmvuy9
My Twitter: https://twitter.com/Machine01776819
Is there a source for this: “AI is used to select which part of the image is best as a preview (for max engagement)”? This is in the Twitter image preview section. I didn’t realize this was a thing and I’d love to learn more about how it works.
I’m a bit upset I can’t restack images because that first meme is 🔥