The Company that changed AI in 2022 [Not ChatGPT or OpenAI]
With all the insane developments in Machine Learning, there is one company that has made developments that will define the field.
Here is an interesting question for you- What company did the most to establish itself in the arms race we have seen going on in Machine Learning?
Most people would think of ChatGPT, which grabbed the attention of everyone. Surely ChatGPT and OpenAI were clearly the most influential companies of last year. If you are among the YouTubers starting AI startups in 10 Easy Steps with ChatGPT, or the ones that are making 10K per day, this would be true for you. However, for the rest of us, things are slightly different (take a look at this breakdown of the issues that ChatGPT and other language models have). In terms of impact on the AI space and how decisions will shape the future, there is one company that made waves in 2022. The decision this company made went unnoticed by many commentators, but it will be a great factor in the Machine Learning space going forward.
So what company am I talking about? And what is this decision they made? Read on.
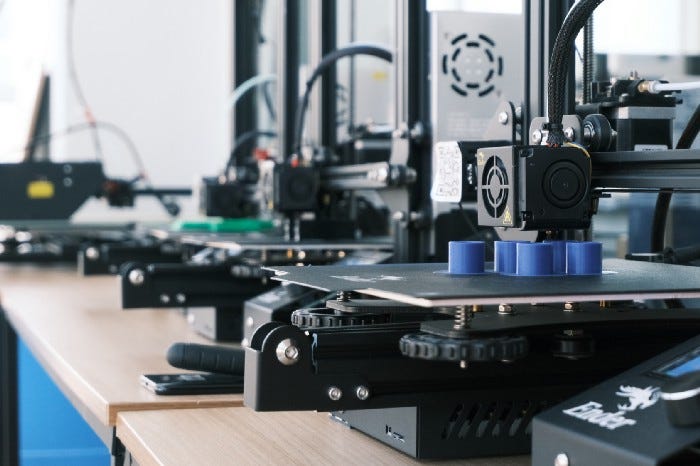
The Company that changed Deep Learning and Data Science
Around the early May of 2022, Meta AI recently released Open Pretrained Transformer (OPT-175B), “a language model with 175 billion parameters trained on publicly available data sets”. While this might seem like another big company joining the LLM wars, the way they did it was a shock in the Machine Learning Community. In their post, Democratizing access to large-scale language models with OPT-175B, Meta had the following to say
For the first time for a language technology system of this size, the release includes both the pretrained models and the code needed to train and use them.
And Meta has continued onwards with their commitment to open source. They have continued to push out language models and ML Research completely open source. In my post about free ChatGPT alternatives, 3 of the models that I covered were models created by Meta AI.
When it comes to future impact, this decision will shake up the domain a lot more than the more trendy topics that captured headlines. For example, while ChatGPT is certainly impressive, it is by no means revolutionary. The capabilities that ChatGPT showed have been showcased by various other models. However, the consequences of Meta’s decision to push for open-sourcing their work will have a lot of implications throughout the field. Let’s cover what they are and how they impact various stakeholders.
Important Talking Points
This decision affects several stakeholders in different ways. Here are a few-
Researchers/Other people looking to learn from this.
Meta itself
OpenAI and other LLM sales companies
The ML/Software Development industry.
Educational/Research Impact
This is a huge win for researchers or anyone looking to learn about Machine Learning. Most notably, this is the antidote to the replication crisis in Machine Learning. I have covered AI’s replication crisis in this article. However, to give you a nutshell, much of Machine Learning is impossible/impractical to reproduce and verify. When it comes to the big companies- like Facebook, Google, and Microsoft- much of this occurs because they are able to train models at a scale that no one else can replicate.
This becomes a problem since it makes it impossible for outside people to break down their findings and find flaws in their methodology. It also severely limits the amount of meaningful discussion we can surrounding a paper/finding when you can’t dig into the nuances of the setup for it.
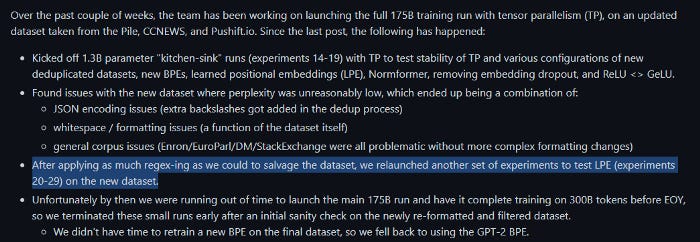
However, that is not all that makes this a big win for Machine Learning Education. When Meta released their code, they also released a lot of other resources. These resources detail the various facets of their large-scale system. My personal recommendation is to read through their Chronicles of OPT-175B training. They detail a lot of the challenges they went through as they were training at this insane scale. Take a look at the following section
It’s been really rough for the team since the November 17th update. Since then, we’ve had 40+ restarts in the 175B experiment for a variety of hardware, infrastructure, or experimental stability issues.
The vast majority of restarts have been due to hardware failures and the lack of ability to provision a sufficient number of “buffer” nodes to replace a bad node with once it goes down with ECC errors. Replacement through the cloud interface can take hours for a single machine, and we started finding that more often than not we would end up getting the same bad machine again. Nodes would also come up with NCCL/IB issues, or the same ECC errors, forcing us to start instrumenting a slew of automated testing and infrastructure tooling ourselves
Taken from their log, Update on 175B Training Run: 27% through
This was an amazing decision taken by the Meta AI team. Reading through these has been interesting, and for anybody who wants to get into Large Scale Deep Learning, understanding their challenges is a must. From a research/education perspective, this decision is a huge win.
Impact on Meta
The impact of this on Meta is going to be harder to evaluate. Releasing this model in the way allowed them to really gain a lot of positive publicity. And the model being released for free also means that people are now much less likely to use paid models from their competitors. This is an edge by itself.
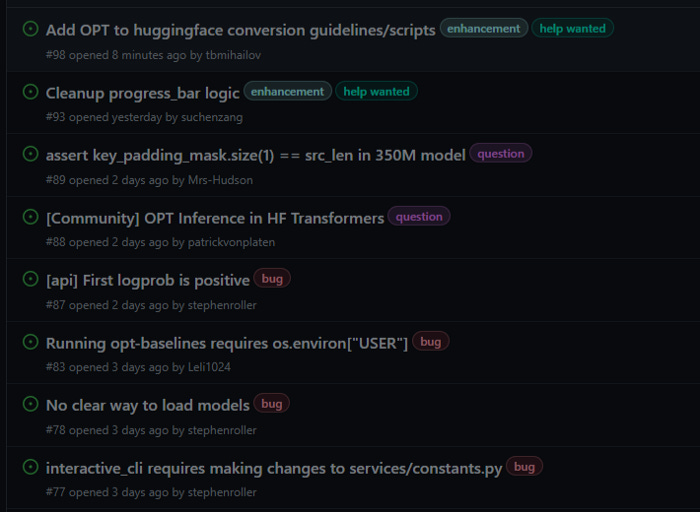
This process also has two other notable advantages. Firstly, since the model is open, it is possible for people to find and discover areas for improvement. This facet of the open-source culture is what is responsible for the explosive growth of tech over the last 2 decades. This gives them access to potentially millions of hours of free debugging/testing done by the community. And they get a lot of insight about what facets the ML community finds the most important/engages with the most.
The second advantage is familiarity with the Meta tech and tools. This is something that a lot of people overlook. Let’s take the example of Tensorflow, by Google. Most serious ML practitioners are proficient with it. This makes it easy for Google to hire ML engineers since most developers will be familiar with the tech. The amount of resources they need to spend training new engineers thus goes down drastically.
Meta opening up all their tools and insights funnels people into the Meta ecosystem of tools and technologies. For their metaverse aspirations to succeed, Meta needs people that are intimate with the frameworks and tech stacks that will be used to build out the platform. Having people develop apps using their technology makes it easier to onboard developers onto this ecosystem.
All of these are huge positives. However, this is offset by a huge problem. Training such a model was extremely costly. To give the whole thing away for free will have a lot of financial implications. While it puts a damper on Open AI and their monetization of GPT-3, it is also going to make it harder for Meta to monetize such a model in the future. However, Meta was wildly profitable last year, so perhaps the pros outweigh this.
Impact on Open AI and other Model Sellers
This is a huge L for Open AI. We have already covered how this will take away a large chunk of the potential customers. It seems like Meta AI has decided to pick a fight with Open AI products. Between Make-A-Scene, their work modernizing CNNs to match Vision Transformers, and OPT, we see a lot of recent releases being competitors to Open AI products.
We developed OPT-175B with energy efficiency in mind by successfully training a model of this size using only 1/7th the carbon footprint as that of GPT-3
The rise of ChatGPT has also come with a large number of startups and companies which are essentially trying to sell ‘foundation models’. The existence of large models, created by a reputed company like Meta, will definitely create a huge pain for these companies. Their decision to open out all their models and logs will act as a strong pull for the
The industry
The Machine Learning industry is definitely licking its lips at this development. For the reasons already mentioned, this is a huge win for AI researchers and developers. This is indirectly a win for the industry.
There are two ways that this situation can play out-
Other tech companies join this trend and they start undercutting each other to gain an edge in the market. Economics tells us that this is amazing for consumers (us).
Business as usual in the industry. The other big companies don’t take the bait. For all the reasons mentioned earlier, this is already a huge win for consumers.
What is often lost when we cover important Machine Learning research is that most of the industry consists of small to medium-sized companies/groups solving very specific problems. While this puts pressure on the big tech companies, this is overwhelmingly a win for the smaller companies, since they get to learn from and use the insights generated from these massive companies without having to churn through the resources themselves. Therefore, this is a huge win for the industry as a whole.
For Machine Learning a base in Software Engineering, Math, and Computer Science is crucial. It will help you conceptualize, build, and optimize your ML. My daily newsletter, Technology Made Simple covers topics in Algorithm Design, Math, Recent Events in Tech, Software Engineering, and much more to make you a better Machine Learning Engineer. I have a special discount for my readers.
Save the time, energy, and money you would burn by going through all those videos, courses, products, and ‘coaches’ and easily find all your needs met in one place.
I am currently running a 20% discount for a WHOLE YEAR, so make sure to check it out. Using this discount will drop the prices-
800 INR (10 USD) → 533 INR (8 USD) per Month
8000 INR (100 USD) → 6400INR (80 USD) per year
You can learn more about the newsletter here. If you’d like to talk to me about your project/company/organization, scroll below and use my contact links to reach out to me.
Reach out to me
Use the links below to check out my other content, learn more about tutoring, reach out to me about projects, or just to say hi.
If you like my writing, I would really appreciate an anonymous testimonial. You can drop it here- https://docs.google.com/forms/d/1Oco7l3A-rE6Ao4E0mB2hgwO6W4nWXwQz3sCa_IYQM5s/edit
To help me understand you fill out this survey (anonymous)
Check out my other articles on Medium. : https://rb.gy/zn1aiu
My YouTube: https://rb.gy/88iwdd
Reach out to me on LinkedIn. Let’s connect: https://rb.gy/m5ok2y
My Instagram: https://rb.gy/gmvuy9
My Twitter: https://twitter.com/Machine01776819