The rise of AI as Magic[Thoughts]
An analysis of the hype around AI and how it causes problems for future adoption
Hey, it’s Devansh 👋👋
Thoughts is a series on AI Made Simple. In issues of Thoughts, I will share interesting developments/debates in AI, go over my first impressions, and their implications. These will not be as technically deep as my usual ML research/concept breakdowns. Ideas that stand out a lot will then be expanded into further breakdowns. These posts are meant to invite discussions and ideas, so don’t be shy about sharing what you think.
I put a lot of effort into creating work that is informative, useful, and independent from undue influence. If you’d like to support my writing, consider becoming a premium subscriber to my sister publication Tech Made Simple to support my crippling chocolate milk addiction. Use the button below for a lifetime 50% discount (5 USD/month, or 50 USD/year).
Executive Highlights
Over the last week, we have seen several notable executives from the AI space make comments about AGI, sparking new hype and speculation on its impact and imminence. At this point, I have spent multiple articles discussing the business motives behind AI Misinformation, some actual AI Risks, how corporate interests perfectly predict the stances of various organizations on the risk from Open Source Foundation Models, and why AGI is never coming. Despite efforts from various experts to quell the hype, the conversation about super AI risks, AGI, and AI manifesting consciousness has only grown.
The increasing interest in Generative AI comes with a greater desire to fit the technology to business problems. Organizations these days spend hours every week in brainstorming meetings about how AI can be used to ‘disrupt the preexisting processes’. This single-minded obsession can lead to some problems.
In the following article we will discuss the following ideas-
How AI has replaced Magic- Many projects and initiatives have fallen into the trap of thinking that AI can magically solve their problems, without giving much thought to the technical challenges. Gen AI is expected to work- no matter what context, data, or budget- through the magic power of 🌟🌟friendship and vibes🌟🌟. This approach has jumped from the private sector (where companies were burning their or their investors’ money) to the public sector, where this pursuit of magic realism takes money away from public programs, consumer protection (financial fraud is on the rise globally, FYI), or reinvestment into public infrastructure.
The externality of this hype-driven thinking- Hype cycles impact real people. Unfortunately, these people are often not considered when making decisions (we are terrible at thinking about the externalities of our decisions). We will cover how reckless decision-making and present business practices can disproportionately impact marginalized communities.
How AI Hype can distract from real issues- To illustrate we will do mini case studies on how Tesla used the AI Robot to distract from business malpractice and faulty results, and California’s attempt to solve traffic with AI and how both divert from deeper, more fundamental problems.
If such ideas interest you, continue reading.
How AI Became Magic
Back when I first got into Machine Learning, the opaque nature and great performance of Deep Learning had led to a very interesting modus operandi- a belief in the general superiority of Deep Learning and the conviction that it would eventually replace every other kind of Machine Learning.
The rationale was relatively simple- we had trained DL models on both vision and text; two notoriously difficult variants of unstructured data. Combined with the dominance of AlphaGo, it seemed like it was only a matter of time before it took over all things related to our lives. To many teams (and venture capital firms), Deep Learning was the silver bullet. As long as you could reframe your problem as a prediction and get some data, it was only a matter of time before your models got good enough to solve it.
Since then, we have learned a lot more about DL, and what it can and can’t do. Far from replacing the grungy parts of AI, DL systems have come to rely on them (Deepmind’s recent AlphaGeometry is a great example of this). This crushed the hopes and dreams of many ML Engineers and Data Scientists who were hoping to make ‘model.fit()’ the entirety of their job description, but we still need to pay for the original sin somehow.
Because language models excel at identifying general patterns and relationships in data, they can quickly predict potentially useful constructs, but often lack the ability to reason rigorously or explain their decisions. Symbolic deduction engines, on the other hand, are based on formal logic and use clear rules to arrive at conclusions. They are rational and explainable, but they can be “slow” and inflexible — especially when dealing with large, complex problems on their own.
-Source. This is the setup I’ve been recommending for months.
Recent conversations around Gen AI have taken much of the same spirit. Instead of treating it as the means to an end, we now see the integration of Gen AI as a meaningful milestone by itself. As if GPT will give you ways to improve your margins by “adding more revenue streams and streamlining operations” that your 400 USD/hour McKinsey suits or Private Equity people wouldn’t give you (AND GPT will give you boring old text, instead of pretty ppts).
Nowhere is this clearer than in the conversations about AGI and its oncoming AI Extinction Risk. Let’s take a look at the steps that we would need to cross to get to that stage-
AI will manifest some kind of general intelligence (keep in mind we haven’t defined this yet) through our training methods. This is a huge assumption, especially when we consider the fact that current training is hitting massive walls at scale, and training on AI-generated data leads to ‘models going mad’.
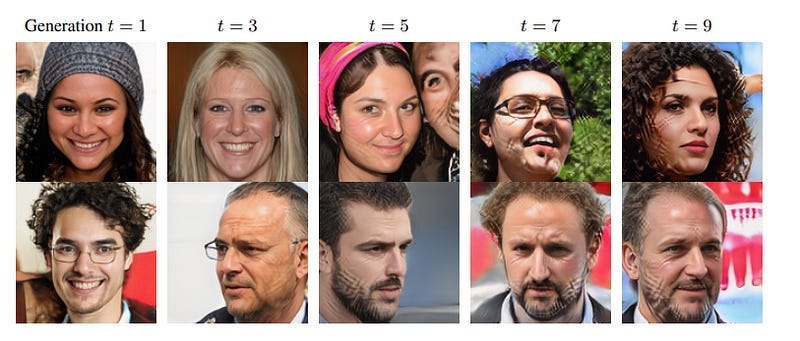
It will gain the ability to perceive both our ‘real world’ and its world created by the training data/sensory input, and use that to act meaningfully in ways. It would need to discard all irrelevant information, and pick correctly b/w conflicting information (if I tell Android 16 that I am a duck, it should not believe me).
Our AGI will have a deep understanding of all the data and the concepts it needs, which will be used to adapt its goals to new circumstances on the fly (any agent that powerful, will noticeably shape its environment, so it must be able to adapt it’s goals accordingly). This is a giant ask when we consider that LLMs haven’t even demonstrated an understanding of language yet.
AI will decide that its best interest lies in harming humans (either directly or indirectly) and act on this.
It will gain the ability to do all of this w/o triggering any flags whatsoever.
Once this happens, we will be unable to stop it or only stop it at great costs. This includes an inability to communicate with the AGI (which would be hilarious when you think about all of this starting from chatbots).
Think about the giant leaps we need to get from one stage to the other and tell me that doesn’t read like magic. Just as we have hypnotized ourselves into believing that Gen AI will magically solve our business problems, another sect have got themselves tripping out over the possibility of us avvarara-cadabraing intelligence into a machine. I recently saw this meme from a prominent Twitter Meme Page (AI Safety Memes)-
The messaging here (both of the meme and the UN Chiefs speech, where he puts AI on the same level of problem as Climate Disaster) is fascinating for what it says about our current society. AI Risk is ill-defined (at least the ones referenced here), hinging on the behavior of misaligned and malicious super-entity. Climate Risk has already manifested as massive weather deviations. When you’re in a rich country like America, that equates to a warm summer and fewer places to go skiing. But when you’re in the Global South- this means massive crop failures, forced migrations, and infrastructural overload.
This is an issue that is often missed in all this euphoria of massive AI Arms races. Meta’s decision to open source their ‘AGI’ is great but the amount of energy it would take to get there is enormous. Not to mention the data waste: the environmental cost of storing data inefficiently.
In 2016, the average business saved and stored 347.56 terabytes of data, according to research from HubSpot. Keeping that amount of data stored would generate nearly 700 tons of carbon dioxide each year.
-Source. Companies trying to outcompete each other in Gen AI will spike this number to the mooooon 🚀🚀.
That’s not all. “In fact, generating an image using a powerful AI model takes as much energy as fully charging your smartphone, according to a new study by researchers at the AI startup Hugging Face and Carnegie Mellon University.” Imagine the cost of deploying multi-modal models to large-scale inference.
And these models would require constant retraining/realignment to stay relevant. And lots of R&D to keep the guardrails relevant( this is amongst the reasons Naval Ravikant’s idea of training an AI Model on all the medical papers will not create the best AI Doctor, as he hoped. Maybe some doctors can add to that one). Think about how quickly we will watch these emissions spiral out of control.
In all such cases, the product user and provider are both shielded from the worst outcomes caused. They get to enjoy productivity gains, adulations for being cutting-edge, and improved valuations. The negative outcomes are passed onto the class of NPCs known as the “Poor Folk of Third World Countries”. And do we really care about them?
Take farmers from my home country, India. They struggle heavily with crop failures as weather patterns shift(especially since many of them rely on monsoons and natural water). These compound other issues leading to massive debts, that cause a lot of anguish and mass suicides. I can’t think of a single time when I took my actions while considering how they would affect the farmers ( or any of the other poor NPCs).
In six years (2015–21), the country lost 33.9 million hectares of the cropped area due to floods and excess rains and 35 million hectares due to drought, which are likely to intensify as various studies predict.
-A year of extreme weather events has weighed heavy on India’s agricultural sector
I can’t speak of anyone else, so I’ll talk about myself. No matter how many poems my school(s) made me recite with the theme ‘Hamara Kisaan Hi Bharat Ki Shaan’, I talk more with Rich Americans than I do with Indian Farmers. I couldn’t name 3 farmers off the top of my head. They are strangers to me. Their lives, challenges, suicides, their very existence is nothing more than a statistic to me. And more often than not, I choose to put my attention to other statistics— one with more immediate and direct relations to me.
This is the problem with externalities. Turns out we really suck at visualizing abstract scenarios and thinking about the downstream impact of our actions. All of our cognitive biases stop us from taking stock of situations and evaluating the risks of our actions on other people(risk expert Filippo Marino has a lot to say on this topic, so I’d suggest following him for the deets). When people make bold claims about AI Risk, they can often draw attention from the very real, risks attacking societies right now.
Let’s end by covering two situations, where AI Hype will lead to worse outcomes for society in more immediate terms. I came across these two developments over the last week that prompted me to write this piece.
AI Robot Smoke Screens and the Eternal Recurrence of Traffic
As mentioned in the summary, there were two very interesting developments over the last week relating to AI and Society. Firstly, we had the government of California decide to use Generative AI to reduce traffic and reduce road safety.
The California Department of Transportation, teaming up with other state agencies, is asking technology companies by Jan. 25 to propose generative AI tools that could help California reduce traffic and make roads safer, especially for pedestrians, cyclists and scooter riders.
This reminds of monorails, hyperloops, self-driving cars, and other futuristic techno-magic that were all hailed to bring a revolution to transportation. Millions of dollars later, they never lived up to the hype. The kicker, this took away from investments into actually proven solutions.
To be clear, I’m not saying AI can’t help with road safety. Back in 2020, I worked with a post-doc at an Indian University (IIT Mumbai) in analyzing the determinants of road mortality to identify high-risk zones/factors. However, this differs meaningfully from Cali’s initiative in two ways-
This wasn’t Gen AI. Somewhat pedantic, but I feel compelled to stress that there are other kinds of solutions in the world. The mentality of trying to fit a solution to a problem is very ultimately wasteful. It will lead to product-market mismatches, such as we saw with Zillow and OpenDoor (highly recommend this video analysis of real-estate tech. It’s 31 mins long, but very worth it).
This project had clearly defined scopes, goals, and gameplans. And it was overseen by experts in the transportation industry. This stops price creep, bloating, and keeps the teams aligned on their goals.
The setup of a project will eventually be reflected in its execution. Vague, unclear, and mismatched initiatives will lead to solutions that pay lip service to technologies as opposed to meaningfully solving problems. I’m not from California, so I can’t comment on the government’s competence, but this is a pattern that has manifested across projects and cultures.
Let’s move on to another major AI news story from this week. Elon Musk continues to perform a massive u-Turn on his AI Alarmism, by presenting a humanoid robot, with the end goal of making “a machine that can replace a human.” His hypocrisy aside, an interesting question to ponder is why? What does he gain from this?
The answer is (shockingly) money. Musk’s companies live and die by his public perception. This is what allows Tesla’s stock to trade at multiples that would be high for tech companies, let alone car manufacturers. Elon is an expert at capturing the media attention and diverting it away from very real concerns about Tesla- their controversy about dumping toxic chemicals, EV fragility in the winters, the fact that Tesla might have lied about manufacturing defects with fatal consequences, and the various problems with Cybertrucks.
Elon has a pattern of diverting the news with outlandish claims, actions, and stunts. His callout of Mark Zuckerberg in MMA, last year is a perfect example. That callout distracted from the negative press swirling around Musk’s mismanagement of Twitter and the fallout from his tweets. This robot was a well-timed diversion of the same nature. Between the bot, Altman’s claims that AGI will be here soon, the AI Summit, and more, this robot would add to the noise around AI and let people forget all the other problems with Tesla.
Here AI is used to play on our diversionary obsession with futurism to paper over the ugly cracks of reality. The coolness (and lobbying) of Electric Cars have led to them attracting disproportionate subsidies and investments at the cost of things that actually work. Sure Electric Cars can be useful, but they also come with lots of flaws that can’t be overcome (cars are by their nature inefficient). These technologies of the future are proving inadequate to solve the problems of today. But we continue to fall for the hype, ignore things that actually work, and burn heaps of money (and the planet).
Next-generation AI will come, and it will unlock massive benefits. But to enable it, we need to lay strong foundations. That requires forethought, meaningful experimentation, and careful investment. Otherwise, we’re all going to engage in busy work, attend 30 webinars on ‘Unlocking the Potential of Generative AI’, and diligently record ourselves accomplishing very little. We would have made a lot of noise only to do nothing, making us like the saddest type of human alive- Arsenal fans.
If you liked this article and wish to share it, please refer to the following guidelines.
That is it for this piece. I appreciate your time. As always, if you’re interested in working with me or checking out my other work, my links will be at the end of this email/post. And if you found value in this write-up, I would appreciate you sharing it with more people. It is word-of-mouth referrals like yours that help me grow.
Reach out to me
Use the links below to check out my other content, learn more about tutoring, reach out to me about projects, or just to say hi.
Small Snippets about Tech, AI and Machine Learning over here
AI Newsletter- https://artificialintelligencemadesimple.substack.com/
My grandma’s favorite Tech Newsletter- https://codinginterviewsmadesimple.substack.com/
Check out my other articles on Medium. : https://rb.gy/zn1aiu
My YouTube: https://rb.gy/88iwdd
Reach out to me on LinkedIn. Let’s connect: https://rb.gy/m5ok2y
My Instagram: https://rb.gy/gmvuy9
My Twitter: https://twitter.com/Machine01776819
You raise such good points, Devansh (and thank you for the kind mention, BTW) - your reference to António Guterres’ warning about AI risks at Davos couldn’t be more on point. The ‘magic’ you speak of is a well-understood phenomenon in psychology as much as in the risk analysis and threat assessment world. Horror fiction aficionados are also intimately familiar with it: the monster left to our imagination will always beat whatever writer or CGI creator can come up with.
Rem: Uncertainty=Anxiety
Interestingly enough, one of the few relatively defendable X-risk drivers (the development of super easy-to-produce yet super-deadly biochemical compounds) also seems to be based on very limited/questionable research (this is a terrific piece on the subject: https://1a3orn.com/sub/essays-propaganda-or-science.html )
In hindsight (which could mean even in a few months), we'll see this AI X risk saga as the most notorious and impactful case of the 'availability cascade' - which will make Y2K and the Segway hype look like child's play.
But your point might deserve an even deeper exploration (maybe a dedicated post?) - could this AI X risks hype cycle carry its own larger risks? A giant red herring distracting us from what is actually harming us?
Most of the debate about recent cracks in the liberal democratic world order in this election-heavy 2024 appears to have shifted from (measurable and documented) disinformation, the rejection of expertise, and the adoption of post-truth political strategies to AI - as if all of the former hadn't preceded it.
Similarly, it wasn't a Terminator AI that fueled the US and global vaccine hesitancy or climate denialism. Yet, aside from acute threats like high-yield weapons or pandemic disease, it would be exactly how you would produce a survival and longevity trend reversal and lead our species toward extinction.
…I could go on and on. Again - keep up this great ‘thought’ experiment.
I really like your writing. However, I want to point out an area where you could use some research.
“Climate Risk has already manifested as massive weather deviations. “
No. Climate is not weather. Weather is not climate. A current media fallacy is to blame every weather ’event’ on climate change. That is fear mongering, click-bait, outright wrong and sucessful marketing. It is spread by ideologues with an agenda, who are advancing a narrative. Do not confuse that with science. Weather has caused loss and devastation as long as there have been people.
A good place to learn more is the Honest Broker Substack with Roger Pilke Jr. He is a professor from CU Boulder, who has testified before Congress multiple times. He covers a lot of controversies in clear tems.
Other than that, great piece. Thanks.