How Will Foundation Models Make Money in the Era of Open Source AI?[Markets]
What Investors and Product People Should Know about DeepSeek Part 2: Navigating DeepSeek, Mistral, LLM Moats, and Distribution. And why Hermes Birkin might be the answer
Hey, it’s Devansh 👋👋
In Markets, we analyze the business side of things, digging into the operations of various groups to contextualize developments. Expect analysis of business strategy, how developments impact the space, conversations about current practices, and predictions for the future.
I put a lot of effort into creating work that is informative, useful, and independent from undue influence. If you’d like to support my writing, please consider becoming a paid subscriber to this newsletter. Doing so helps me put more effort into writing/research, reach more people, and supports my crippling chocolate milk addiction. Help me democratize the most important ideas in AI Research and Engineering to over 100K readers weekly. Many companies have a learning budget that you can expense this newsletter to. You can use the following for an email template to request reimbursement for your subscription.
PS- We follow a “pay what you can” model, which allows you to support within your means, and support my mission of providing high-quality technical education to everyone for less than the price of a cup of coffee. Check out this post for more details and to find a plan that works for you.
This is part 2 of our series on DeepSeek and how developments in OpenSource AI will affect the business models for foundation models (part 1 over here). Gen AI has led to productivity increases in many areas, and it’s clear that the potential isn’t fully tapped out yet. Thus, there is a lot of commercial potential in the technology, but people are unsure of how to unlock it- especially given the massive investments and the rise of Open Source models that can match closed models in both quality and speed-
There are some great discussions on how Generative AI will make money, but here’s the thing everyone misses- the commercialization of foundation models is not just an engineering or product strategy question. The final strategy that a company will adopt will as much be driven by internal politics and PR as it is driven by actual business/tech fundamentals.
This conversation gets even murkier when we consider the misunderstood nature of open-source LLMs, whose performance, lower investment, and “free” price leads people to think they will wipe out demand for Closed Models-
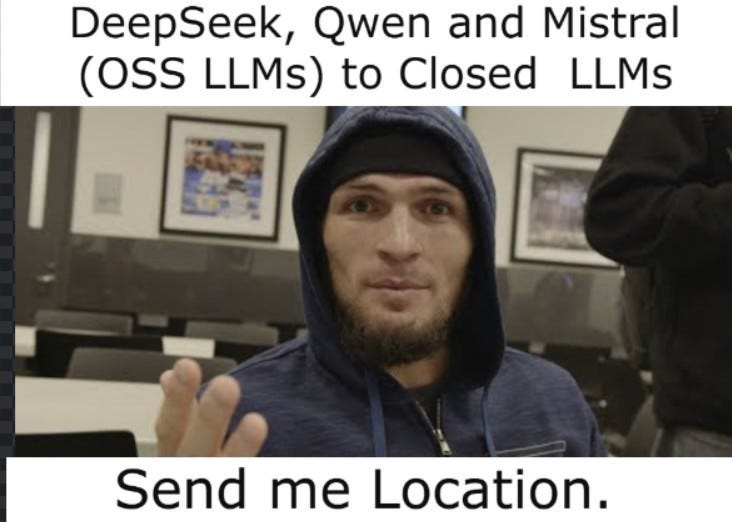
In this article, I will cover the major business models for foundation models to answer the question that is on everyone’s mind- how will Foundation Models like ChatGPT, Gemini or Claude make money, especially in the era of “Free” Open Source Foundation Models?
Spoiler- one business model is inspired by luxury Hand Bags (actual Luxury). Read to the end to find out how Luxury Brands might have the business models that LLM providers should copy.
Executive Highlights (TL;DR)
At the very highest level, we have 2 main ways to monetize LLMs-
Subscriptions.
Subscriptions are how most people currently interact with LLMs. Subs have a simple business model- you pay X USD per month to interact with ‘LLM Y’ Z number of times.
Subs are appealing for a large variety of more straightforward work where people want to interact with the LLM directly- either in single or multi-step conversations. This is best when you need the LLM for relatively simple tasks that can be solved with the LLM’s stored internal knowledge + inputs w/o too much wrangling.
From a business perspective, here is what stands out to me about Subscriptions in LLMs-
Subs have a relatively high upfront upside but capped earning potential (you won’t earn more than your sub cost). This can be a problem since LLM inference is expensive. Netflix can serve subs at scale since it doesn’t cost at that much to provide movies to an additional customer (the major costs in storage, talent, licenses etc are all paid at once). LLM providers have high recurring costs through inference at scale, and capping the revenue can skew unit economics against you.
It’s easiest to sell, but since it has the least customization, it also leads to the lowest “stickiness”. People can drop your premium subscription for another one pretty quickly.
To an extent, it turns LLMs from commodities into apps, which can help with fighting the nasty price wars we’re seeing in the API space (and is common in commodities). However, I think point 2 still makes your overall demand very elastic to price, which make subs themselves very vulnerable to price wars-
All in all, relying on subs creates an interesting tension with your users- you want users who buy your subscription but don’t use it, which will drop your loyalty even more. Otherwise, your options are to cap usage (Claude has usage limits even for paying users) or to potentially eat losses (OpenAI) running your LLMs-
If you think that Subscriptions are the best way forward, you’re making the following bets-
Most tasks are simple enough to be done through a general interface that can mass appeal to audiences.
The Trend of LLM Inference costs going down is “real”- it reflects the costs going down for the providers, not the providers eating a bigger loss to get market share.
“Real” Inference costs will keep going down, increasing the margins of your subscription. For example, if you can bring the real inference cost down to 0.03/million tokens (I’m blending input and output tokens here for simplicity)- then the costs a user could have to run ~66K queries with a size of 10K size to be within bounds (massive input corpora and additional tools like search/citations can wipe this difference very quickly, but this is still a big number), with a 20 USD subscription. However, these are some very favorable numbers (Gemini 1.5 8B, by far the cheapest good model, is 0.3/MTok output and 0.075/MTok input, which is still quite a bit more expensive than this).
Imo- 1 is true to an extent, 2 is nonsense, and 3 is possible but has substantial technical and political challenges holding it back. That’s why I’m not bullish on them. I’ll talk about them in a separate piece, but here is a quick overview-
Many teams have political/branding reasons to keep pushing scaling. This was my only real issue with Recursion Pharmaceuticals, for example. While I’m largely positive about their approach, I think it’s a pretty big red flag that they repeatedly sell their investments in a Super Computer w/o talking about any meaningful business evals on why this was better than alternatives(I flagged this when I covered them). To me, this seemed like yet another company using the Deep Learning + Nvidia + Scaling Hype to drive up valuations (a notion they seemed to take offense to, but never directly countered). This is not unique to RXRX. There are several times when teams choose more expensive approaches, like scaling over the more beneficial approaches (see this for a detailed list), because of misaligned incentives. The sunk costs, talks around model scale, etc all will make it very hard for anyone to ask for huge changes.
Technically, we have several optimizations around LLMs, which might make switching to more efficient setups difficult.
I’m not a journalist or a gossip blogger, so I don’t really write about the conversations I have with senior leaders in Big Tech who tell me all kinds of horror stories about the pushback they receive for trying to optimize things by going in new directions. But these pressures are stronger than you’d think and will stop the real costs from going down too much.
API
This is my favorite way of monetization. LLM providers provide API keys that allow builders like myself to pay per token usage. The upfront returns of this are low, but API-based billing has some benefits-
The returns are not capped.
API-based builders spend more time optimizing their product around an LLM and its peculiarities and face a higher opportunity cost for switching. This forces better loyalty.
Fewer memory/security concerns storing all the conversations- which, as DeepSeek showed us, isn’t as trivial as it seems-
Within minutes, we found a publicly accessible ClickHouse database linked to DeepSeek, completely open and unauthenticated, exposing sensitive data. It was hosted at oauth2callback.deepseek.com:9000 and dev.deepseek.com:9000.
This database contained a significant volume of chat history, backend data and sensitive information, including log streams, API Secrets, and operational details.
More critically, the exposure allowed for full database control and potential privilege escalation within the DeepSeek environment, without any authentication or defense mechanism to the outside world.
API pricing currently faces a huge problem since it turns LLMs into commodities (raw materials used by other products for value adds), opening them to price wars. Since commodities are very tied to price, this leads to massive price wars and low margins for everyone involved. This is why estimates say that LLM API margins have been dropping-
I like the business of Margins of APIs because of the eventual upside. It feeds the naturally monopolistic nature of tech, and any provider that achieves meaningful technical differentiation will take the market. While this will not happen in the short-medium term (the space is still very young), the returns when it eventually does it will be massive. That end makes this worth playing for.
To put it another way, I like APIs because, in my mind, competition is largely irrelevant when you become the best at what you do. APIs become massively lucrative when you hit this stage. If I were leading an LLM provider, I would keep my sights firmly on this goal. Shying away from this fight is playing to avoid defeat, not playing to win, which is a very wimpy way to mark your existence on the planet.
Most LLM providers will play with these offerings in different ways to create their monetization plans (the major ones already offer both subs through chat interfaces and LLM APIs). However, they can go in a lot of directions from here. The main article will cover the following-
How can LLM providers engage in vertical integration- either in chips/LLM inference, moving to the application layer, providing services, or partnering with providers (mimicking Palatir)? This will open new revenue streams, cut costs, and allow better product adoption.
Why LLM providers might want to take a cue from the massively lucrative fashion industry to create gated access. This gated access will improve model security and position LLMs as a premium good. Sounds silly, but this has worked for the High Fashion industry, and I think it might be an interesting approach.
Navigating LLMs to Profitability in the Era of Powerful Open Source Models.
Keep reading if that interests you.
I put a lot of work into writing this newsletter. To do so, I rely on you for support. If a few more people choose to become paid subscribers, the Chocolate Milk Cult can continue to provide high-quality and accessible education and opportunities to anyone who needs it. If you think this mission is worth contributing to, please consider a premium subscription. You can do so for less than the cost of a Netflix Subscription (pay what you want here).
I provide various consulting and advisory services. If you‘d like to explore how we can work together, reach out to me through any of my socials over here or reply to this email.
How LLMs would benefit from Vertical Integration
Next up would be vertical integration. Here the model providers will expand either backward or forward in the AI Value Chain. Backwards will cut costs while going forward will add revenue streams-
There are a few ways this can show up:
LLM Providers Go into Hardware-
See Sam Altman, Amazon, and Google all trying to build chips to serve AI Models more efficiently. This drops their reliance on external providers like Nvidia AND gets better performance since these chips are specialized for AI Models-
Someone buys LLMs-
This is Google’s model with their Gen AI (or it was supposed to be if that company wasn’t filled with generationally talented at fumbling it’s technical lead). While most people know that Gemini is a good revenue generator for Google Cloud, they don’t know that Google has a few other AI offerings (such as a not great, but still functional) video embedding model that’s only available through Google Cloud. The playbook for such an approach is simple- use LLM to draw people in and then upsell them into your ecosystem.
Google is a good case study for those interested in this play. They had all the pieces all the way back in early 2022- with a high-performing multimodal Model (PaLM) and the TPUs for efficient inference. However, their awful product strategy, marketing, sales, and terrible Google Cloud platform all massacred what was a massive technical lead-
Anyone looking to copy this playbook must be careful to not repeat their mistakes.
Applications:
Cue Meta. Meta doesn’t profit from LLMs directly, but does need them to ensure that their platforms can run better. They have made a lot of money through their open-source strategy since they were able to crowd-source the improvement of Llama, which they were able to leverage. Instead of trying to monetize LLMs directly, providers can let the Open Source movement improve their existing offerings, and then fold those offering back into their offerings that leverage LLMs.
Services:
Lastly, LLM companies can move towards providing services to implement AI-based products. This allows them to add additional revenue streams (both from servicing and ensuring that their products are used) and tap a large market of non-technical high-ticket spenders with lower churn- governments, law firms, finance firms etc. There are 3 flavors of services that can be done
Accenture et al- Use your brand to get big contracts, either execute on them or sub-contract them out. Big 4 consulting companies have shown the benefit of such an approach, booking record revenues by selling their “expertise” to charge big bucks for stupidly simple software. If something goes out of their depth, they can always hire more specialized firms for a portion of the billing to make money w/o having to work themselves. That’s arbitrage baby.
However, copying this playbook isn’t for most Silicon Valley players, who have a superiority complex towards services firms (there is a belief that products are inherently more lucrative, which I think is a myth based on extrapolation from a few winners and incomplete). For people who want to stay in the product lane, but start monetizing services, the next playbook might be good.
Palantir and Forward Deployment Engineers- Palantir is a very good demonstration of how lucrative it can be to combine products and services by onboarding high-ticket clients onto their products. To ensure the best customer service/acquisition, they have an army of trained forward deployment engineers -engineers who interact directly with their customers. This business model has worked very well for them-
LLM providers can replicate this by partnering with existing service providers (who have deep relationships with local governments) and training their workforce to act as the forward deployment engineers and taking advantage of their relationships for quicker adoption. This is a win win- providers get into new customers w/o doing much legwork, and the consulting companies get to upskill in relevant technologies and get better projects based on their relationship with the provider.
If there are any AI Labs or Providers that want to break into the NYC governments, give SVAM a spin. We’ve got some pretty deep relationships and a good team that would require minimum training. Email me- devansh@svam.com, if you want to talk.
Cohere: LLM provider Cohere takes this strategy a step further. Along with the above methods, they straight up sell their LLM to people that want a powerful LLM on-premises. This strategy is less sound now that we have powerful Open Source LLMs. Cohere is still alive b/c of their strong DevRel and Open Source work, but they have fallen off pretty hard from the conversations I’ve had. I don’t think this is a viable primary strategy, but it could be a way to monetize some of the older/unpopular LLM projects that people want to buy for reverse engineering purposes.
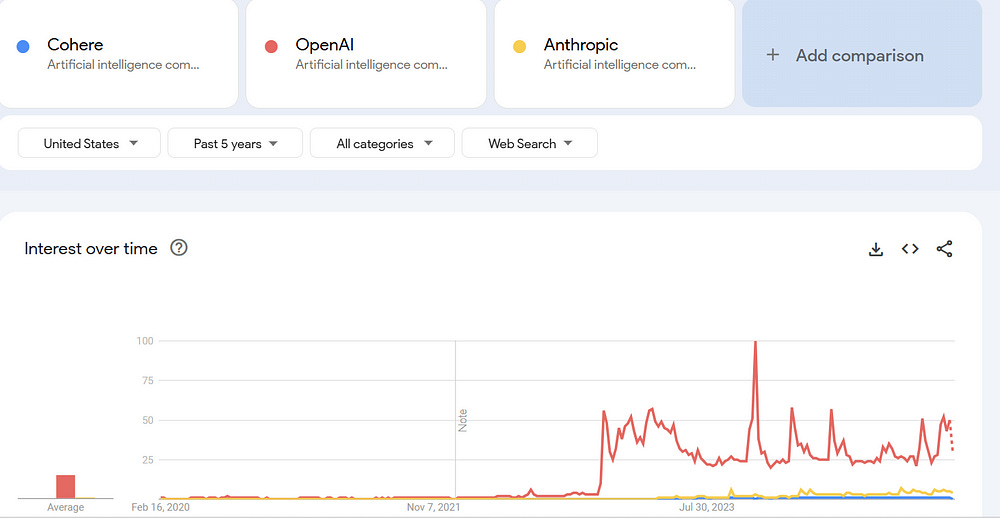
This covers the here and now/. But what about the Future?
How Gated Access and Partnerships might be the Foundation Model Business Model of the Future
Complete credit for this thought goes to my friend and top investor, Eric Flaningam.
With technologies like Model Distillation that can remove technical advantages b/w Foundation Models, certain providers might be hesitant to provide access to the full API to unchecked users. This is where the Gated Access Strategy can become useful. Here, an LLM provider might sign a minimum-use contract with an enterprise customer to allow access to their best LLM. This LLM will only be available to certain customers who have spent a certain amount of money on the other offerings, essentially becoming a luxury good.
While this seems like a very strange idea, there are some pretty good business reasons why this might work -
This guarantees revenue from the minimum usage contracts.
People underestimate how powerful the weaker models are for most tasks. Many tasks can be done by building on them.
This ensures that the most powerful LLMs are safe from copying, since their usage can be tracked more easily.
Branding is a moat, and this positions the LLM partnerships as a desirable trait. There is a certain desire to have what other people have, even if it’s useless to us. This plays on that perfectly.
The higher-tier LLMs can be priced accordingly, unlocking better margins.
The more I think about it, the more I’m convinced that this strategy is money. Turning the best LLMs into status symbols will make the users much less likely to switch to competitors. The only drawback of this will be that the “best” LLM will also be the least tested, which can be a huge concern for anyone who wants to deploy it right away. However, this can be mitigated by providing the LLM in the Subscription Chat interface, giving people exposure, and collecting detailed feedback while limiting exposure. This will also drive up usage of the chat platform, creating some synergy b/w offerings.
Those are all the major business models that are viable for LLM providers. I expect LLM providers to blend the various approaches to find their sweet spot. As mentioned in the API section, I think LLMs are a naturally monopolistic section where the best provider will take the market. While we don’t know who or how, this is how most technology plays out as it matures. Any LLM provider/investor would do well to make moves with this fact in mind. Just as Yama approaches the dying on his Buffalo, the march of Tech toward consolidation might seem slow, but it is in the periphery, steadily gaining ground on us. To that end, it is best to anticipate this consolidation and act accordingly.
If I may, I would like to end with a small note to the leadership of the LLM providers with my advice on how they should navigate this space, given the relentless pressure from the Open Source community.
How to have Profitable Generative AI in the Era of “Free Open Source”.
AKA why LLM Providers should not be Scared of Open Source
Recent events have revealed an interesting irony- many LLM leaders, traditionally seen as champions of the free market and deregulation, have recently gone begging the US government to lobby strong export controls to stop DeepSeek, to protect “US interests”. This isn’t the first time LLM Providers have been spooked by Open Source (Sam Altman famously testified for the importance of regulation for AI when Llama was first leaked, and “Safe AI”/extinction risk from LLMs has been one of the most prominent Silicon Valley shams meant to fear monger and capture regulation.
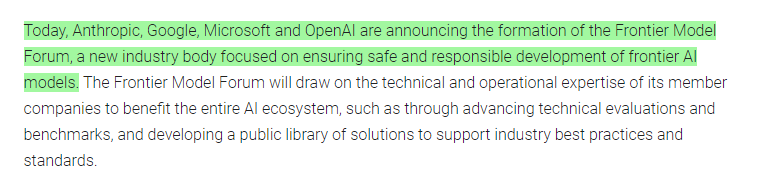
I want to end this article with a simple plea to these bastions of freedom and progress. Instead of running away from the Open Source movement, embrace it. Your fear of the Open Source movement hurts not just other people but, most importantly, your own profit margins.
Firstly, Open Source is good for innovation. While people who don’t understand the business of technology think this cuts your margins, any deep study will show you the opposite. OSS leads to more innovation, and as people at the forefront of the tech with heaps of resources, you can leverage it better than anyone else.
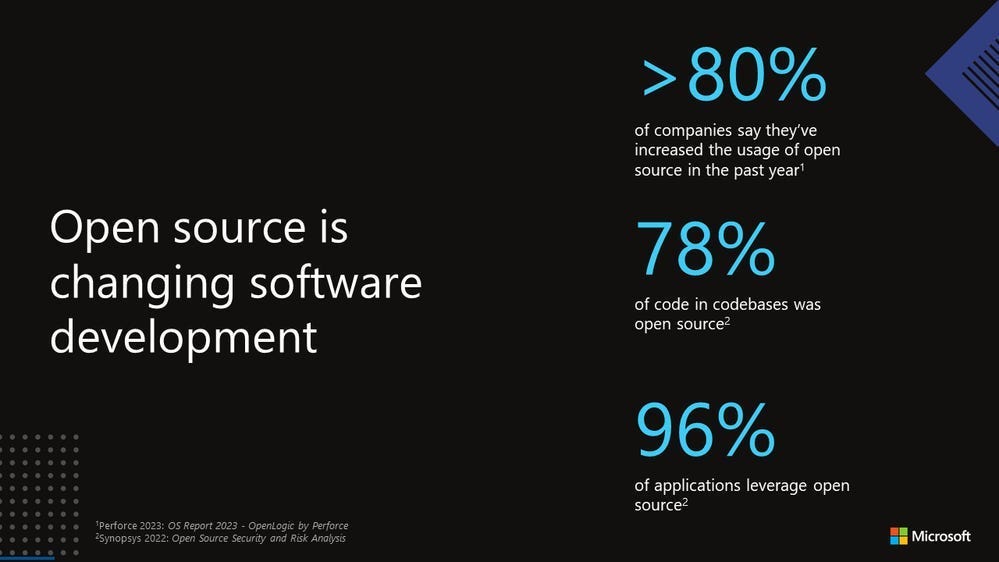
This is particularly important since this will accelerate your progress, allowing you to become the best in the space and letting you profit from the monopoly. By restricting Open Source, you restrict the creation of your monopoly.
Secondly, when you spend all your effort on hype-based misinformation and regulatory capture, you convey a very clear signal admitting defeat. You tell the world you don’t think you can win w/o relying on these other tactics. You tell everyone that your survival hinges on other people down, not in rising above. Noone seems to have yet, but eventually your technical employees will read between the lines and hear what you’re not saying- “You (the employees) are not good enough”. Can’t imagine that this would do wonders for employee morale or productivity.
And lastly, it’s not a good look. Maybe these are just cultural differences since I grew up in New Delhi and not Silicon Valley. In New Delhi, you don’t run away from a fight when someone steps on your turf. Not when you call yourself the baddest kid on the block. And especially not when you’ve had billions of dollars, access to the brightest talents, a government that rigs circumstances in your favor, and every other advantage you can imagine. If you keep running, ducking, and crying to Daddy Trump for more handouts- we wouldn’t call you smart, strategic, or prudent.
In New Delhi, we’d call you a bitch.
Maybe that’s just cultural differences. Happy to be educated if that is the case.
Thank you for being here and I hope you have a wonderful day,
I ended up learning way more about Luxury Brands than I planned to,
Dev <3
If you liked this article and wish to share it, please refer to the following guidelines.
That is it for this piece. I appreciate your time. As always, if you’re interested in working with me or checking out my other work, my links will be at the end of this email/post. And if you found value in this write-up, I would appreciate you sharing it with more people. It is word-of-mouth referrals like yours that help me grow. You can share your testimonials over here.
=
Reach out to me
Use the links below to check out my other content, learn more about tutoring, reach out to me about projects, or just to say hi.
Small Snippets about Tech, AI and Machine Learning over here
AI Newsletter- https://artificialintelligencemadesimple.substack.com/
My grandma’s favorite Tech Newsletter- https://codinginterviewsmadesimple.substack.com/
My (imaginary) sister’s favorite MLOps Podcast-
Check out my other articles on Medium. : https://rb.gy/zn1aiu
My YouTube: https://rb.gy/88iwdd
Reach out to me on LinkedIn. Let’s connect: https://rb.gy/m5ok2y
My Instagram: https://rb.gy/gmvuy9
My Twitter: https://twitter.com/Machine01776819